The Ultimate Guide to Leveraging Predictive Customer Analytics for Improved Retention
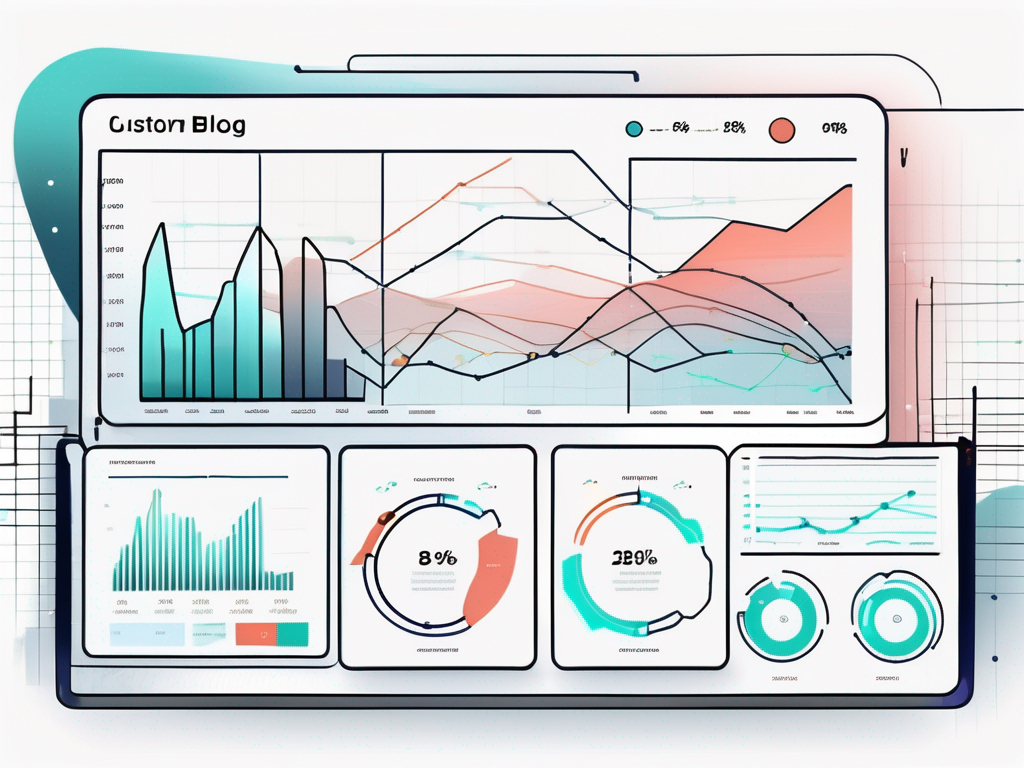
In today's highly competitive business landscape, customer retention has become more critical than ever. Companies are constantly seeking ways to keep their customers engaged, satisfied, and loyal. One tool that has gained significant traction in recent years is predictive customer analytics. By leveraging data and advanced algorithms, businesses can gain valuable insights into customer behavior and make informed decisions to enhance the overall experience. In this ultimate guide, we will demystify predictive customer analytics, explore its pitfalls and benefits, and delve into strategies for leveraging this powerful tool to improve retention.
In a Nutshell
Predictive customer analytics is the use of historical data, machine learning, and statistical modeling to forecast future customer behavior. Businesses can analyze large volumes of customer data to identify patterns, trends, and anomalies, enabling them to make proactive decisions that drive customer retention and satisfaction.
With predictive customer analytics, companies can not only understand their customers better but also predict their future needs, preferences, and actions. This enables proactive and targeted interventions to address potential issues or seize opportunities, ultimately leading to improved customer retention and loyalty.
One key aspect of predictive customer analytics is the utilization of various data sources to create a comprehensive view of each customer. By integrating data from multiple touchpoints such as online interactions, purchase history, social media engagement, and customer service interactions, businesses can gain a holistic understanding of individual customer journeys. This detailed view allows companies to tailor their marketing strategies and product offerings to meet the specific needs and preferences of each customer segment.
Moreover, predictive customer analytics empowers businesses to forecast customer lifetime value accurately. By analyzing past buying behavior, engagement patterns, and demographic information, companies can predict how much value a customer is likely to generate over their entire relationship with the brand. This insight enables organizations to allocate resources effectively, focusing their efforts on high-value customers and implementing retention strategies for those at risk of churning.
Demystifying Predictive Customer Analytics
Decoding Customer Behavior for Future Predictions
One of the key benefits of predictive customer analytics is understanding customer behavior. By analyzing past interactions and purchase history, businesses can identify patterns and trends that can help predict future behavior. This enables companies to tailor their offerings, personalize experiences, and proactively address customer needs.
Spotting Red Flags: Identifying High-Risk Customers
Another crucial aspect of predictive customer analytics is identifying high-risk customers. By analyzing certain indicators such as declining engagement, increased complaints or returns, and changes in purchase patterns, businesses can flag customers who are at risk of churn. This allows proactive measures to be taken to retain these valuable customers.
Elevating Satisfaction Levels and Fostering Loyalty
Predictive customer analytics also plays a vital role in enhancing customer satisfaction and fostering loyalty. By analyzing customer feedback, sentiments, and preferences, businesses can identify areas for improvement and make proactive changes to their products, services, and experiences. This helps in creating a loyal customer base that is more likely to stay engaged and recommend the business to others.
Moreover, predictive customer analytics can also be utilized to forecast market trends and anticipate shifts in consumer behavior. By analyzing data from various sources such as social media, market research reports, and competitor analysis, businesses can gain valuable insights into upcoming trends and consumer preferences. This foresight enables companies to adapt their strategies and offerings to stay ahead of the competition and meet evolving customer demands.
Additionally, predictive analytics can aid in optimizing marketing campaigns and improving customer segmentation. By leveraging predictive models to identify the most effective marketing channels, messaging strategies, and target audiences, businesses can allocate their resources more efficiently and achieve higher conversion rates. This targeted approach not only maximizes the impact of marketing efforts but also enhances customer engagement and brand loyalty.
Pitfalls of Predictive Customer Analytics
While predictive customer analytics offers great potential, it is important to be aware of its potential pitfalls. One common pitfall is relying too heavily on algorithms and automation, neglecting the importance of human expertise and intuition. It's crucial to strike a balance between data-driven insights and human judgment.
Another pitfall is overgeneralizing customer behavior based on past data. Customer preferences and behaviors can change over time, and it's important to continuously update and refine predictive models to ensure accuracy.
Lastly, data privacy and security are critical considerations when using predictive customer analytics. Businesses must ensure compliance with regulations and take appropriate measures to protect customer data.
One additional pitfall to watch out for is the issue of sample bias in predictive customer analytics. When collecting data for analysis, it's important to ensure that the sample is representative of the entire customer population. If the sample is skewed or not diverse enough, the predictive models may not accurately reflect the behavior of all customers.
Moreover, a common mistake in predictive customer analytics is overlooking the importance of qualitative data. While quantitative data is essential for building predictive models, qualitative data, such as customer feedback and sentiments, can provide valuable insights that quantitative data alone may not capture. Incorporating both types of data can lead to more comprehensive and accurate predictions.
Strategies for Data Collection in Predictive Analytics
Harnessing Feature Tagging for Customer Interaction Tracking
Feature tagging involves adding relevant metadata to customer interactions. This enables businesses to track and analyze specific customer behaviors and preferences, providing valuable input for predictive models. By analyzing these tagged interactions, businesses can gain insights into patterns and trends that inform future predictions and decision-making processes.
For example, imagine a scenario where a customer interacts with an e-commerce website by browsing through different product categories, adding items to their cart, and finally making a purchase. By tagging each of these interactions with relevant metadata, such as the product category, price range, and time of interaction, businesses can analyze the customer's journey and understand their preferences and buying patterns. This information can then be used to personalize recommendations, optimize marketing campaigns, and improve overall customer satisfaction.
Unveiling Insights with Product Usage Data and Heatmaps
Product usage data and heatmaps offer valuable insights into how customers interact with products and services. By analyzing usage patterns, businesses can identify pain points, areas for improvement, and opportunities for personalized recommendations. These insights can be used to optimize the customer experience, enhance satisfaction, and drive retention.
For instance, consider a software company that provides a project management tool. By collecting and analyzing product usage data, such as the frequency of task creation, collaboration among team members, and time spent on different features, the company can identify which features are most commonly used and which ones are being underutilized. This information can help them prioritize feature enhancements, streamline user workflows, and ultimately deliver a more intuitive and efficient product.
Gathering Feedback Through Microsurveys for Enhanced Loyalty
Microsurveys are short, targeted surveys that capture customer feedback and preferences. They provide businesses with real-time insights into customer sentiments and can be used to identify emerging trends or issues. By collecting and analyzing this feedback, businesses can make data-driven decisions to enhance loyalty and retention.
For example, a mobile app developer may use microsurveys to gather feedback from users about their satisfaction with the app's performance, user interface, and overall experience. By analyzing the survey responses, the developer can identify areas for improvement, address any bugs or usability issues, and prioritize new feature development based on user preferences. This iterative feedback loop helps build a loyal user base and ensures continuous improvement of the app.
Grouping Customers for Analysis Through Behavioral Segmentation
Behavioral segmentation involves dividing customers into distinct groups based on their behaviors and characteristics. By grouping customers with similar attributes, businesses can analyze and predict behavior patterns more effectively. This enables targeted interventions and personalized experiences that drive customer retention.
For instance, an online streaming platform may use behavioral segmentation to categorize its users into groups based on their viewing habits, such as genres watched, time of day, and device used. By understanding the preferences and behaviors of each segment, the platform can curate personalized recommendations and tailor its content library to cater to different user tastes. This targeted approach enhances user satisfaction, increases engagement, and ultimately boosts customer loyalty.
Trend Spotting Through Historical Data Analysis
Analyzing historical data allows businesses to identify trends and patterns that can help predict future customer behavior. By understanding how customer preferences and behaviors have evolved over time, businesses can proactively adapt their strategies and offerings to meet changing demands. Trend spotting is a powerful tool for predicting and addressing customer needs.
For example, a fashion retailer can analyze historical sales data to identify seasonal trends, popular styles, and customer preferences. By spotting these trends, the retailer can adjust their inventory, marketing campaigns, and product offerings accordingly. This proactive approach ensures that the retailer stays ahead of the competition, meets customer expectations, and maximizes sales opportunities.
Leveraging Predictive Data Analytics for Customer Retention and Experience Enhancement
Personalizing In-App Experiences for Optimal Engagement
Personalization is a key driver of customer satisfaction and retention. By leveraging predictive data analytics, businesses can deliver highly personalized in-app experiences that resonate with individual customers. From personalized recommendations to customized interfaces, personalization enhances engagement and fosters loyalty.
Tailoring In-App Messaging to Customer Preferences
Effective communication is paramount for customer retention. Through predictive data analytics, businesses can understand how customers prefer to be communicated with and tailor in-app messaging accordingly. This ensures that customers receive relevant and timely information, enhancing their overall experience and loyalty.
Preventing Customer Churn Through Proactive Measures
One of the primary objectives of predictive customer analytics is preventing customer churn. By identifying high-risk customers and analyzing patterns that indicate potential churn, businesses can implement proactive measures to retain these customers. Whether it's offering personalized incentives, providing exceptional customer service, or addressing pain points, proactive retention strategies are critical for long-term success.
Driving Product Innovation Through Data Insights
Predictive data analytics not only helps in customer retention but also drives product innovation. By analyzing customer behavior and preferences, businesses gain valuable insights that can be used to develop new features and improve existing products. These insights enable businesses to stay ahead of the competition and deliver products that meet the evolving needs of their customers.
Enhancing Customer Support with Predictive Analytics
Customer support plays a crucial role in customer satisfaction. Predictive analytics can be used to enhance customer support by identifying common issues and providing proactive solutions. By analyzing customer data, businesses can anticipate potential problems and offer timely assistance, ensuring a smooth and seamless customer support experience.
In conclusion, predictive customer analytics is a powerful tool for improving customer retention and satisfaction. By decoding customer behavior, identifying red flags, and personalizing experiences, businesses can enhance their customer relationships and drive long-term loyalty. However, it's important to be aware of potential pitfalls and ensure data privacy and security. With the right strategies in place, predictive customer analytics can be the key to unlocking success in the modern business landscape.
Additional Resources